- Those who have data but lack the analysis skills and don’t know where to start 🤦
- Anyone looking to visualize analytical insights quickly 📈
- People wanting to try real data analysis using sample data and free tools 📖
Do you have data but feel lost about how to analyze it or create meaningful visualizations and reports? Try out HEARTCOUNT’s AI analysis feature, Dialogue. It makes it easy for anyone to quickly gain insights from data.
What is Dialogue?
Dialogue is HEARTCOUNT’s interactive AI analysis tool that performs data analysis like a conversation. You can ask questions and interpret your dataset, and Dialogue will respond with charts, insights, tables, and even suggest follow-up questions for deeper analysis.
In this post, we’ll explore how to use Dialogue by analyzing one of HEARTCOUNT’s sample datasets: the HR dataset.
How to Use Dialogue for AI Analysis
Step 1: Uploading the Dataset
First, log into HEARTCOUNT and you’ll see the option to create a New Campaign
.
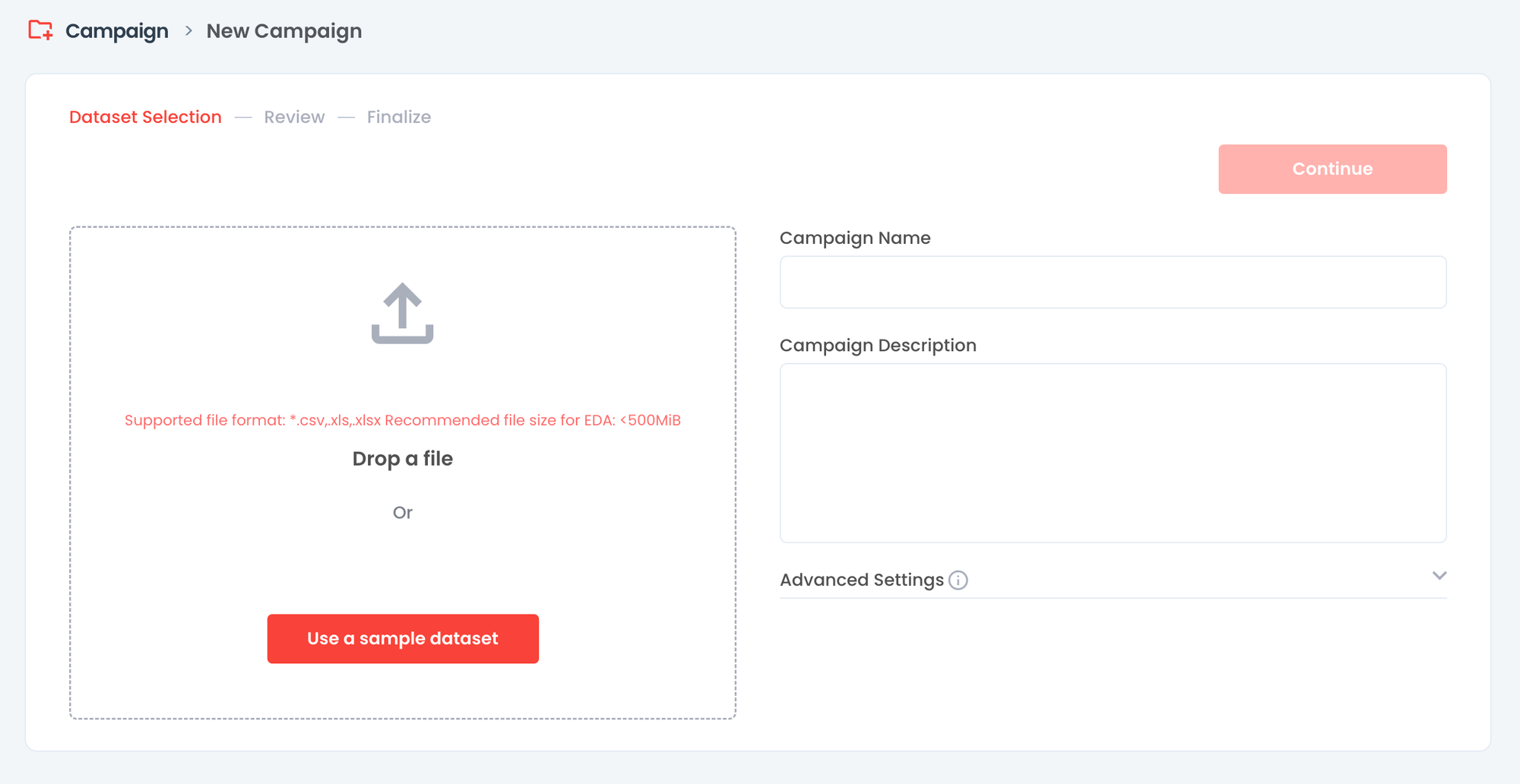
Click on the Use Sample Dataset
button to browse the available datasets, then select the Employee (HR) Dataset
.
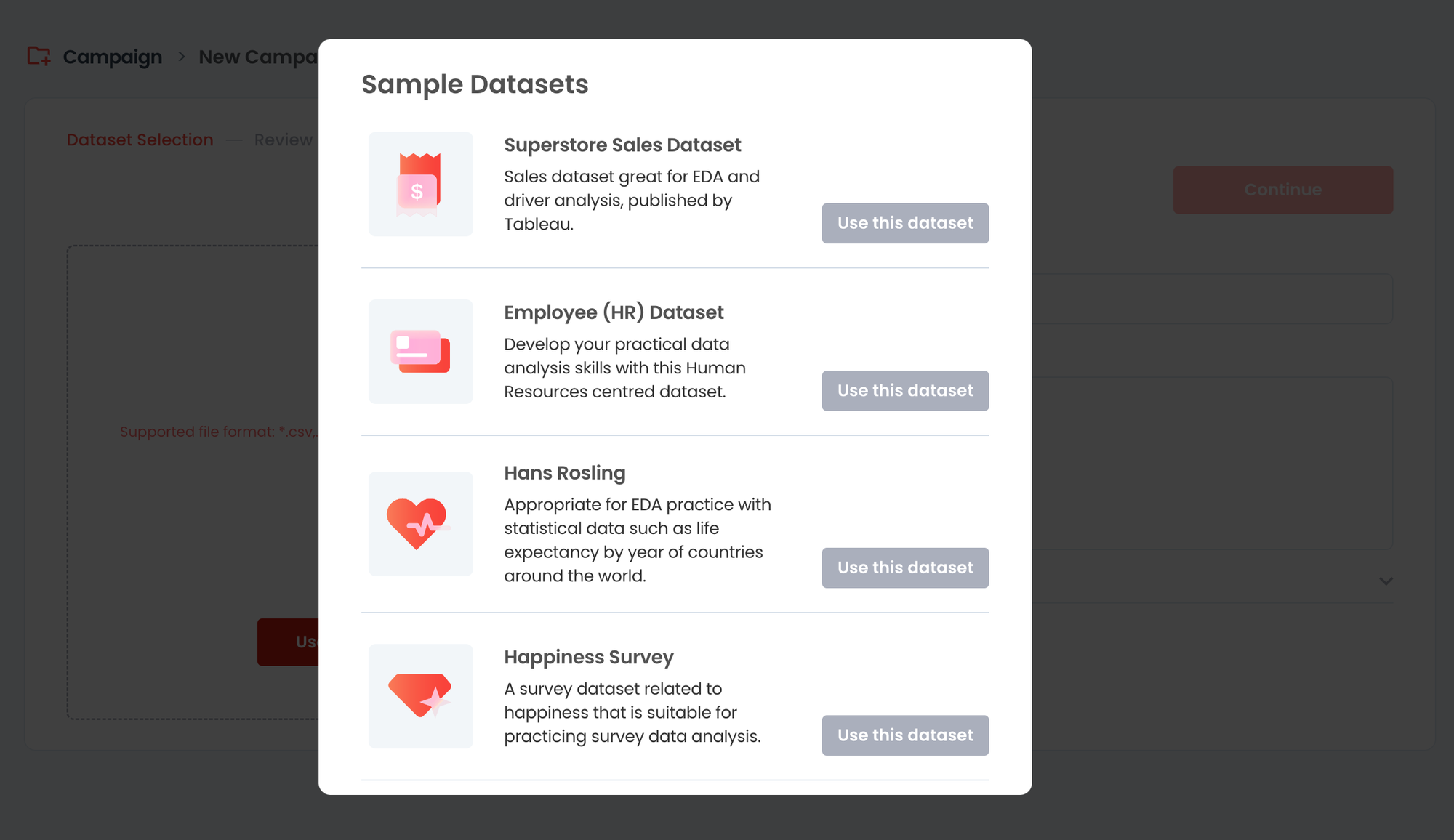
Step 2: Choosing Key Metrics – Let’s Analyze Performance Scores by Hiring Source
Once you access Dialogue, you’ll be asked to choose which variables to focus on. In the HR dataset, the key metrics are Performance Score and Employee Satisfaction (Engagement Score).
Let’s start by analyzing performance scores. Next, we’ll select Hiring Source to analyze performance scores by different hiring channels.
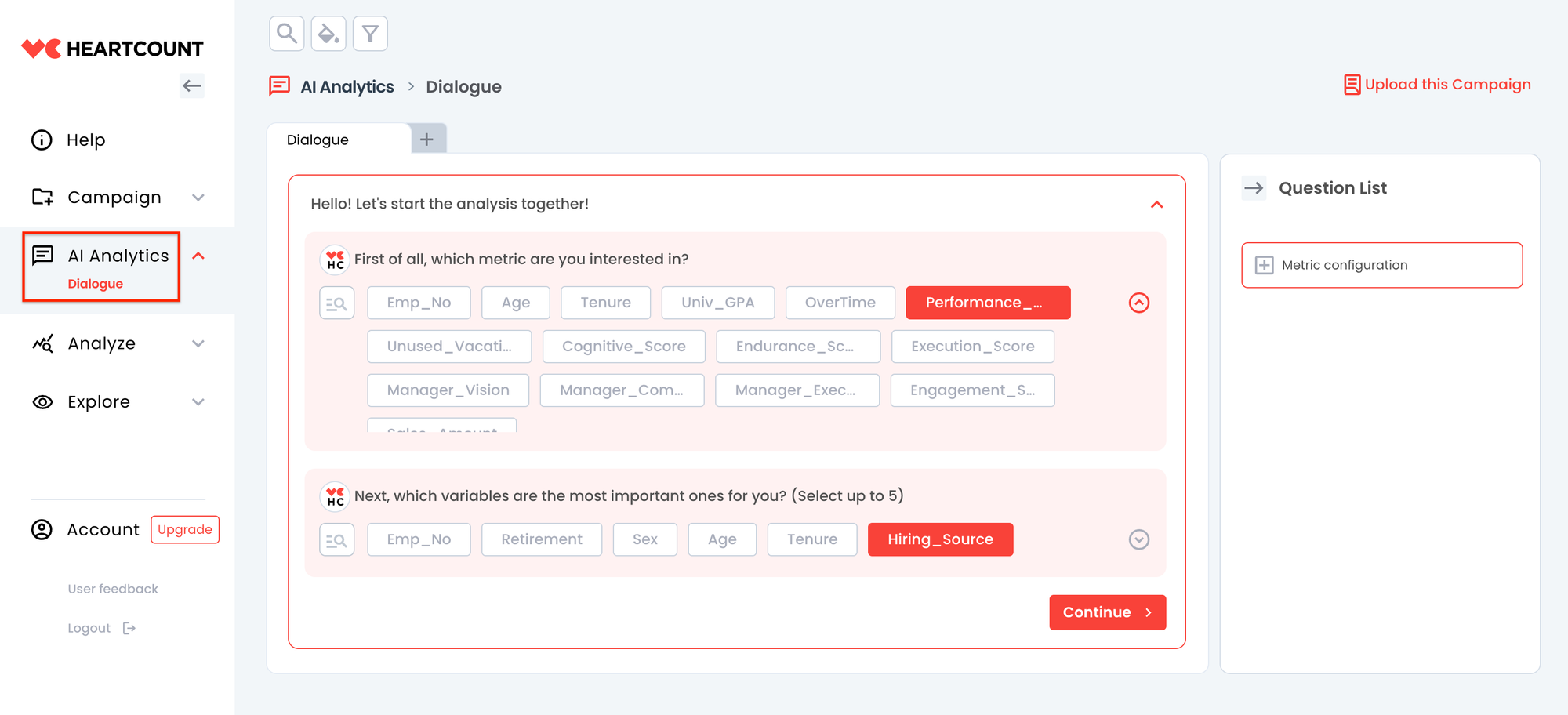
Once set, Dialogue will provide you with a list of relevant questions.
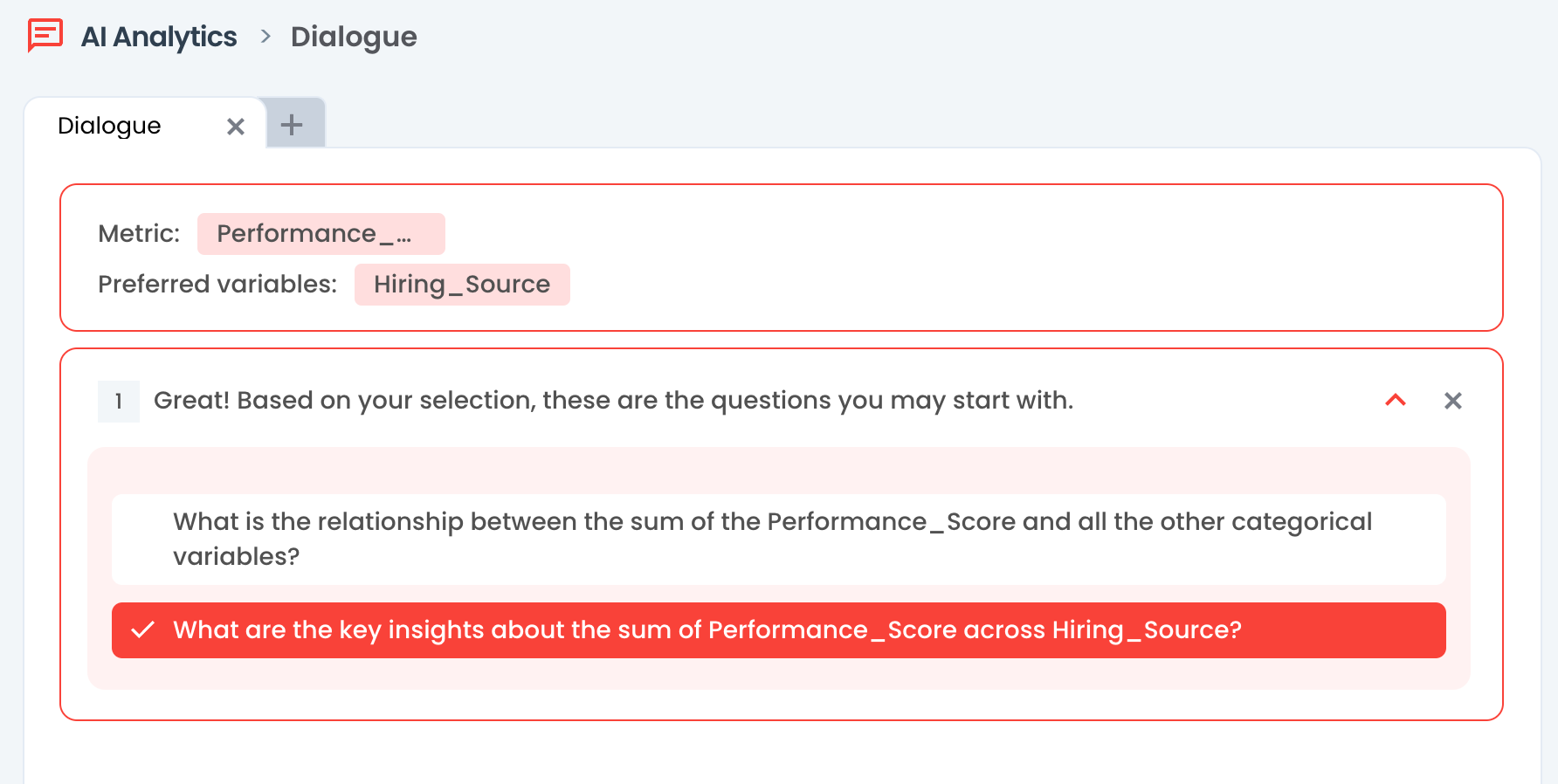
Let’s choose the second question: “What are the key insights about the sum of Performance_Score across Hiring_Source?”
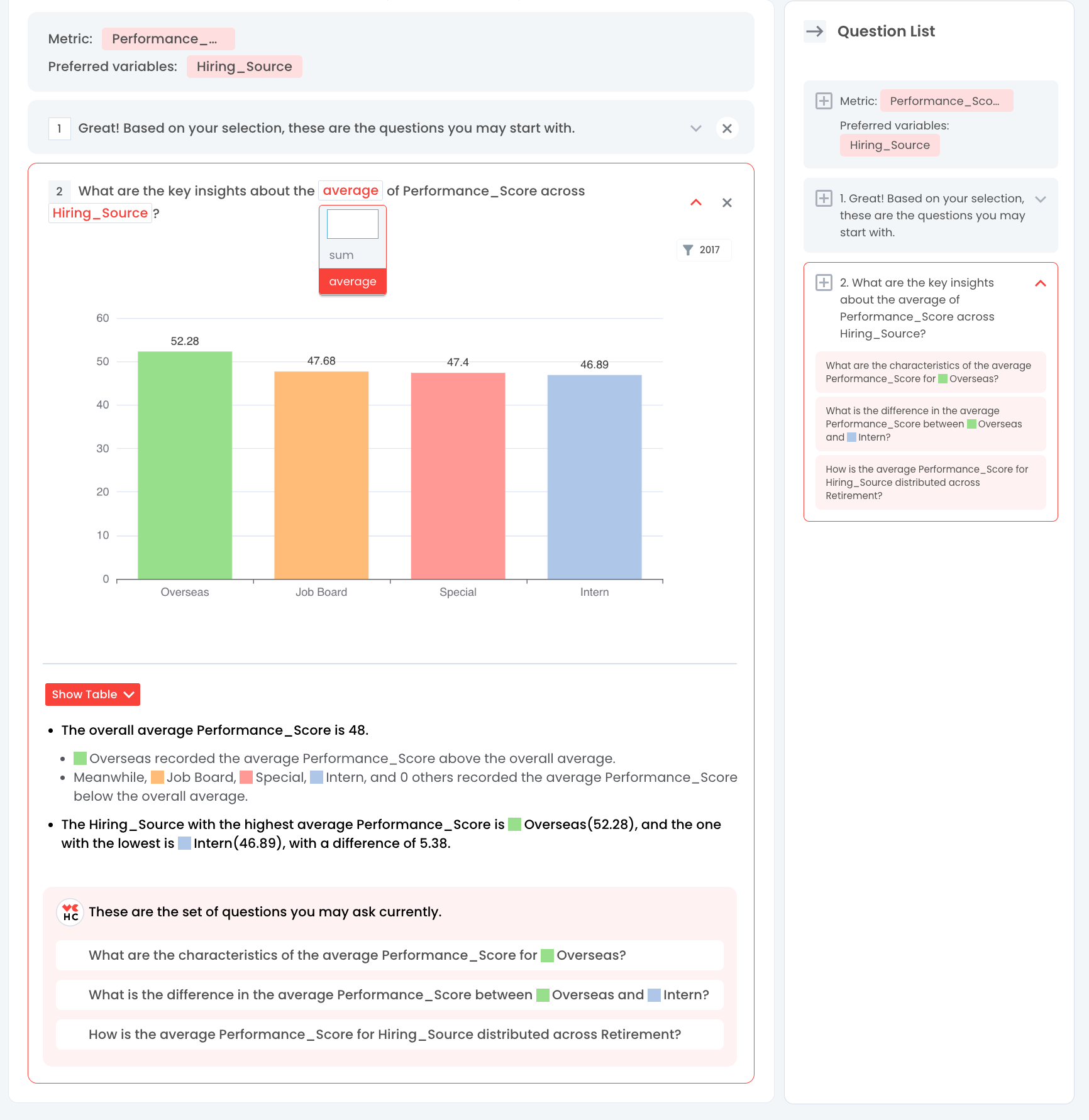
Since the number of employees differs by hiring source, it’s better to look at the average performance score rather than the total. So, we’ll adjust the question to reflect the average.
The results show that employees hired from overseas have the highest average performance score, while interns have the lowest.
Step 3: Analyzing by Location
Now, let’s see if performance scores vary by work location. The overall pattern remains the same: overseas hires outperform, and interns score the lowest. However…
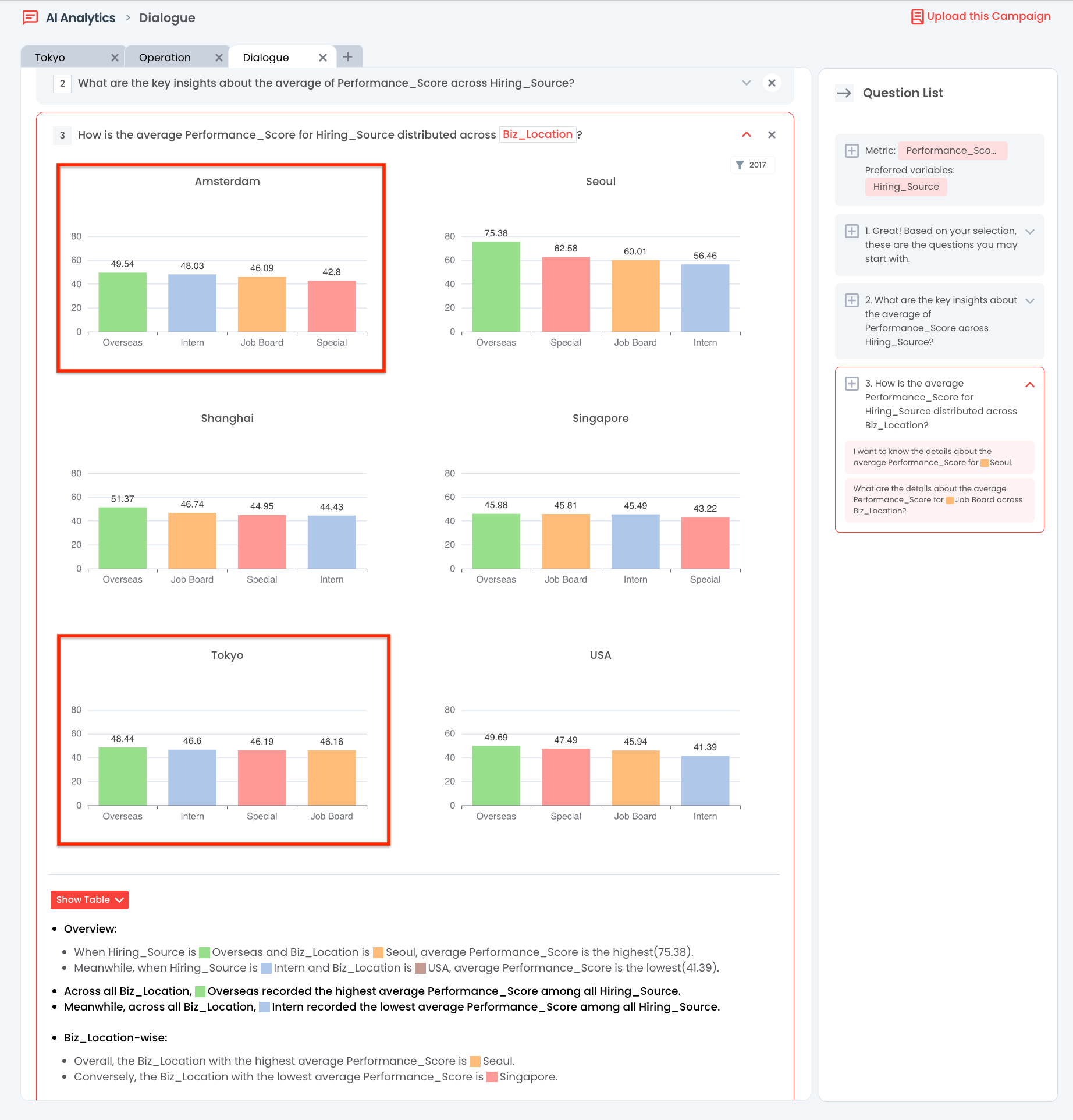
By default, the recommended question is set to focus on Seoul, where the average performance score is the highest among locations. You can select this question and adjust the parameters if needed.
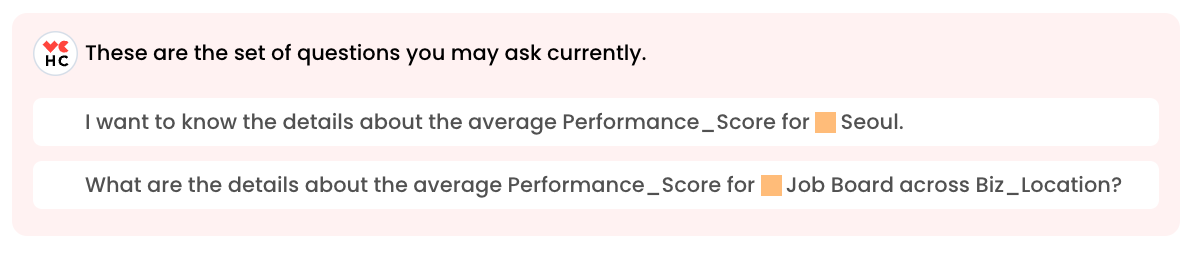
Here, let’s take a closer look at the performance scores of interns in Tokyo. To break down performance scores by region, select the first question and choose Tokyo as the location.
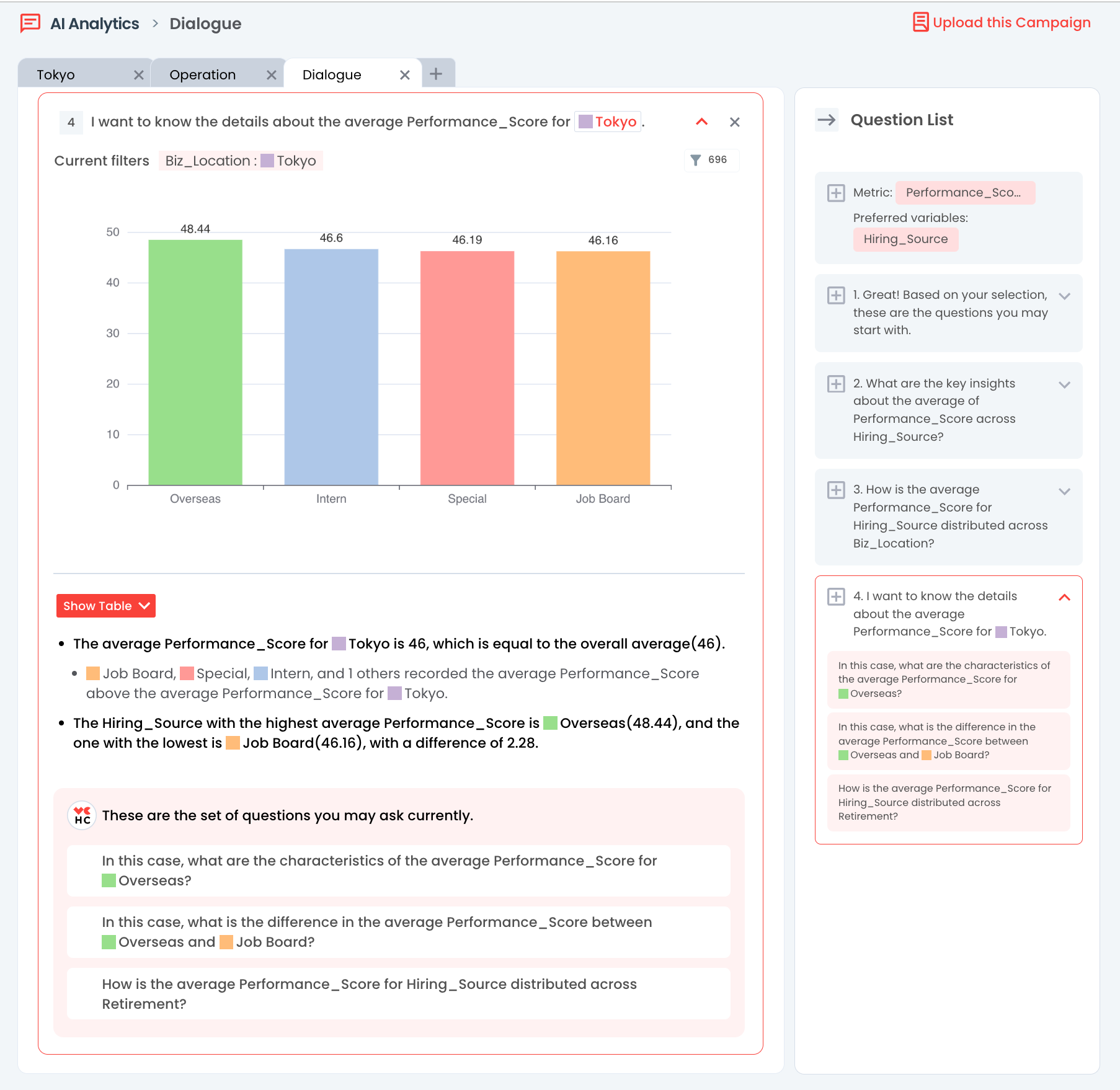
Step 4: Breaking Down by Team
We’ll now explore the performance scores of interns by team. Select Team Division instead of Hiring Source, and focus on Tokyo interns.

- Change Overseas to Intern
- Change Retirement to Team
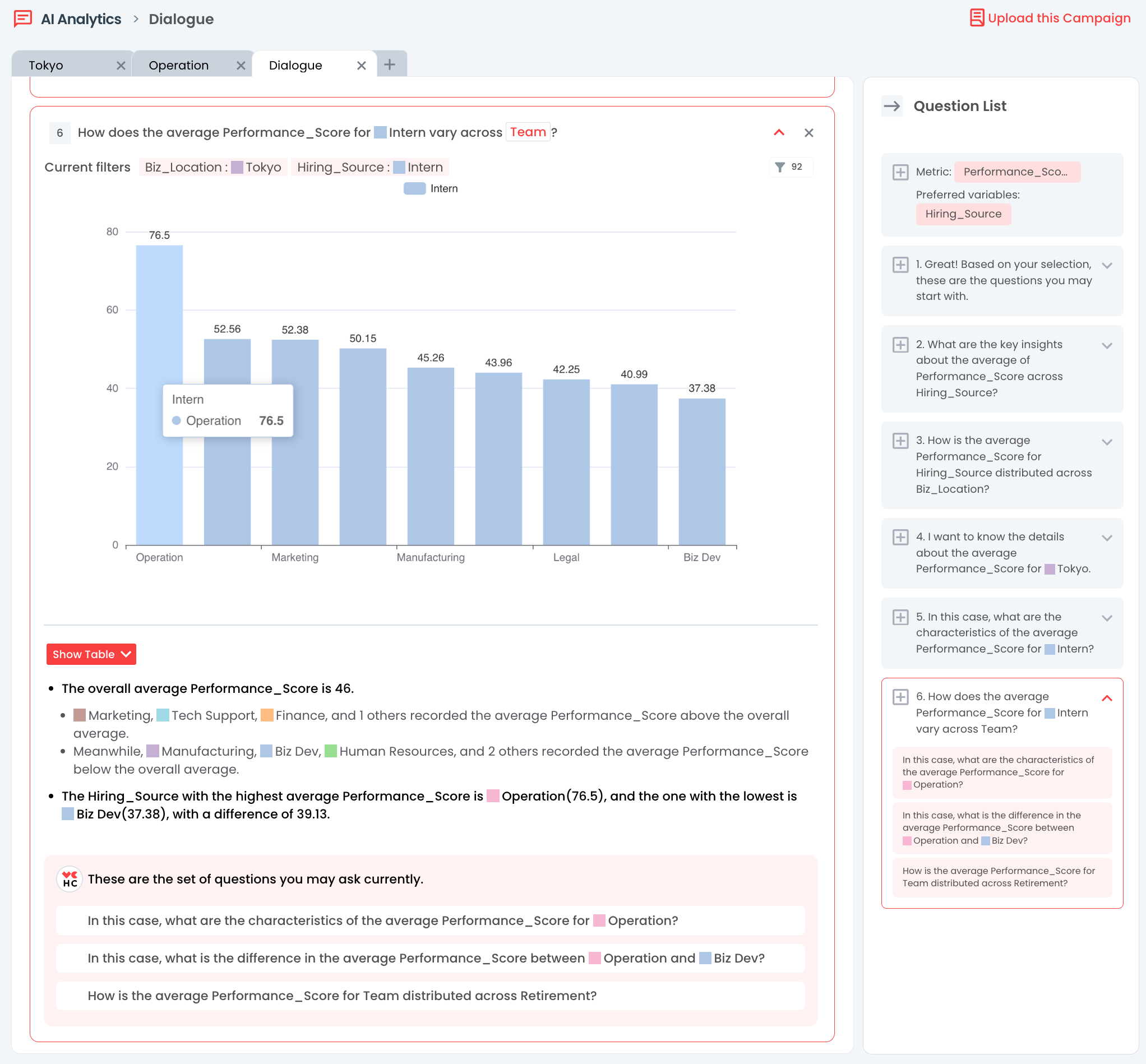
Could the Operations Team’s Performance Score Be Generally High?
However, before jumping to conclusions about the excellence of the Service Operations Team, we need to compare these scores with other regions. If the average performance score of the Service Operations Team across all locations is 80, a score of 76.5 in Tokyo may not be that impressive.
Using the Question List
feature on the right, we can revisit and adjust previous questions.
- Shall we take a closer look at the average performance score by hiring source from the second question?
- Click the plus button on the left to open a new tab and continue the analysis from that point.
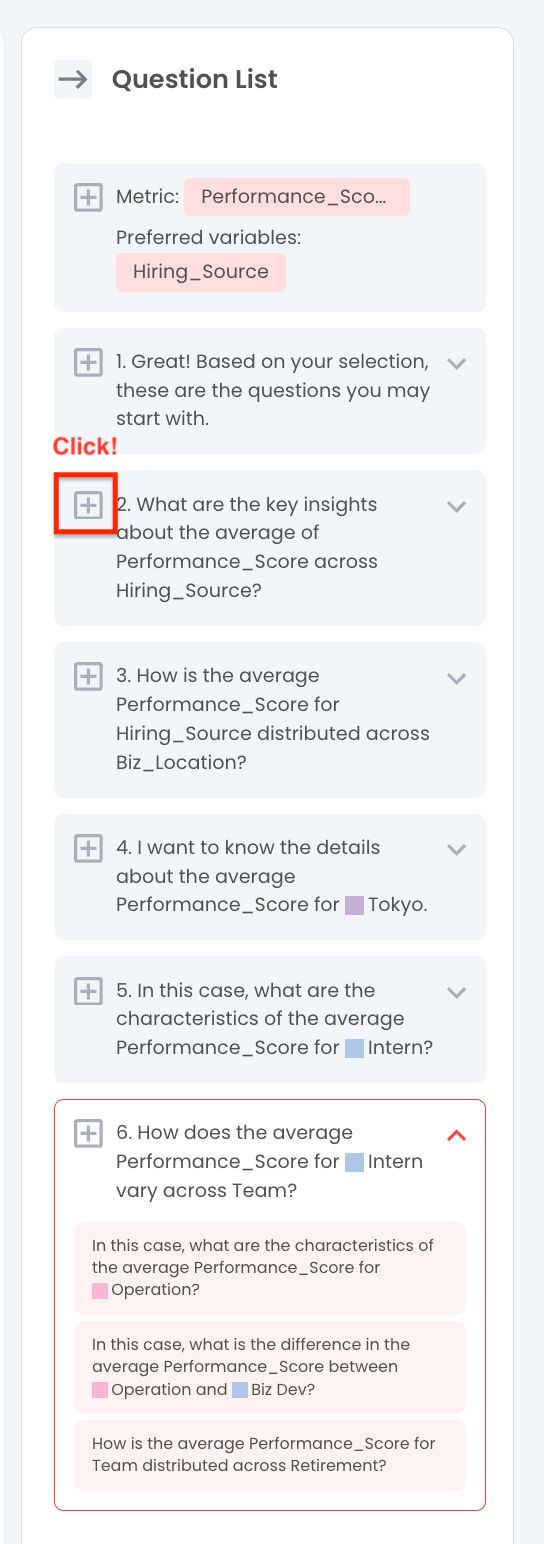
Switch the analysis from Hiring Source to Team Division, and the average performance score of the Service Operations Team across all regions is 65.95.
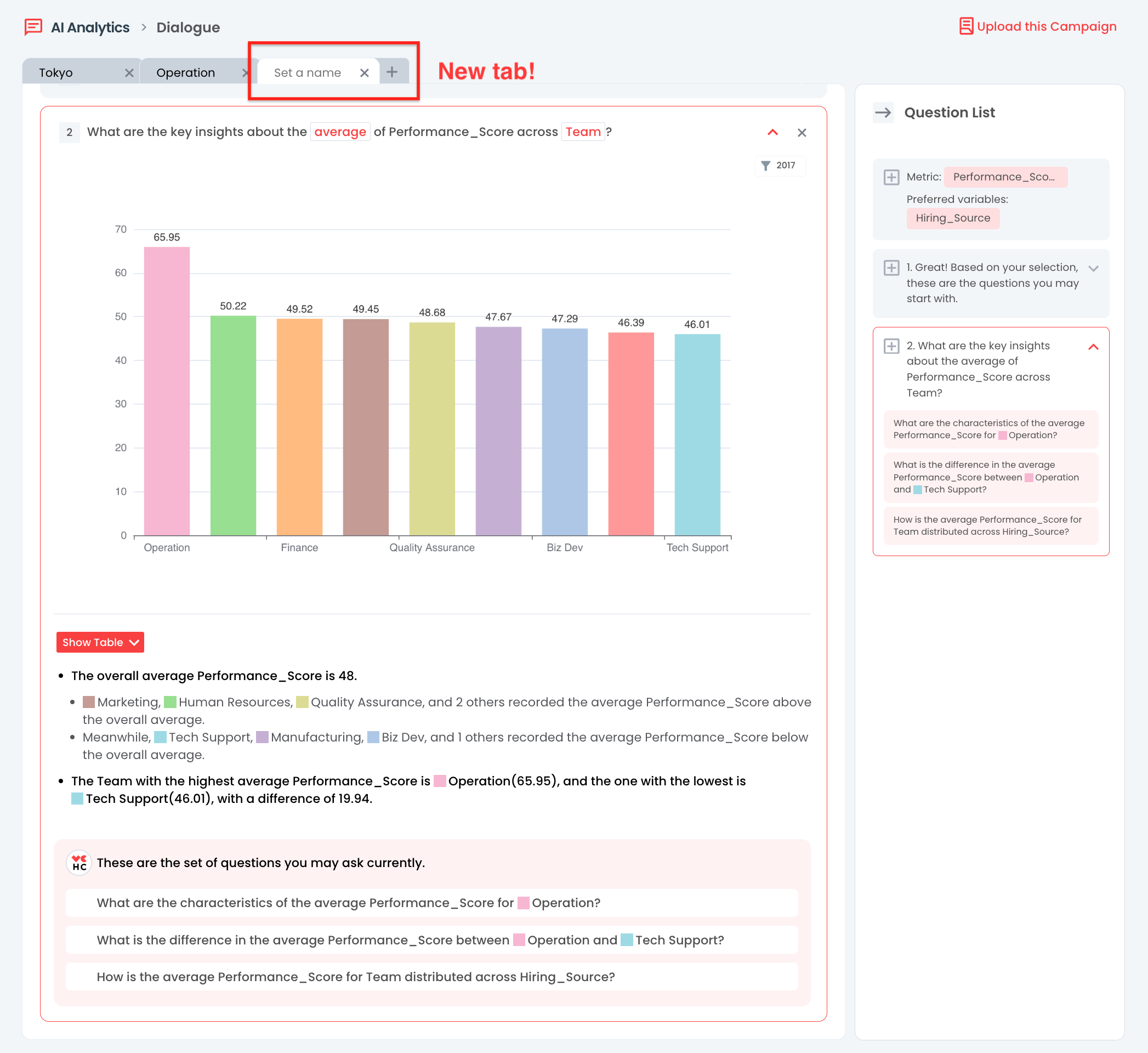
With a sufficient amount of real data, we could further explore in-depth questions like, “Why did interns in the Tokyo region perform better?” or “What factors might have caused this difference?” However, in the sample dataset, the intern data for Tokyo is limited to 92 entries, so we’ll conclude the analysis here.
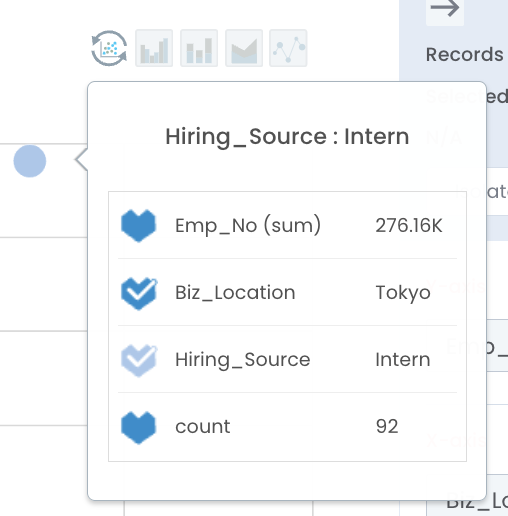
Final Thoughts
We’ve seen how Dialogue helps you quickly uncover insights from data. As a next step, you can try exploring the Employee Satisfaction (Engagement Score) metric and apply the following steps:
• Explore Engagement Score by Job Family.
• Compare the engagement scores of Manufacturing vs. Biz Dev.
• Break down engagement scores by Biz location or Gender.
Analysis Tips
- Which location shows the biggest difference in average engagement score between the two job categories?
- In Amsterdam, how do satisfaction levels compare when we break down the data by gender for each job category?
- For female employees, what new insights emerge compared to what we saw earlier?
As you get more comfortable with Dialogue, you’ll be able to use your own work data to find valuable insights that could be used in this month’s report!
Start for free now.